Integrating wind variability to modelling wind-ramp events using a non-binary ramp function and deep learning models
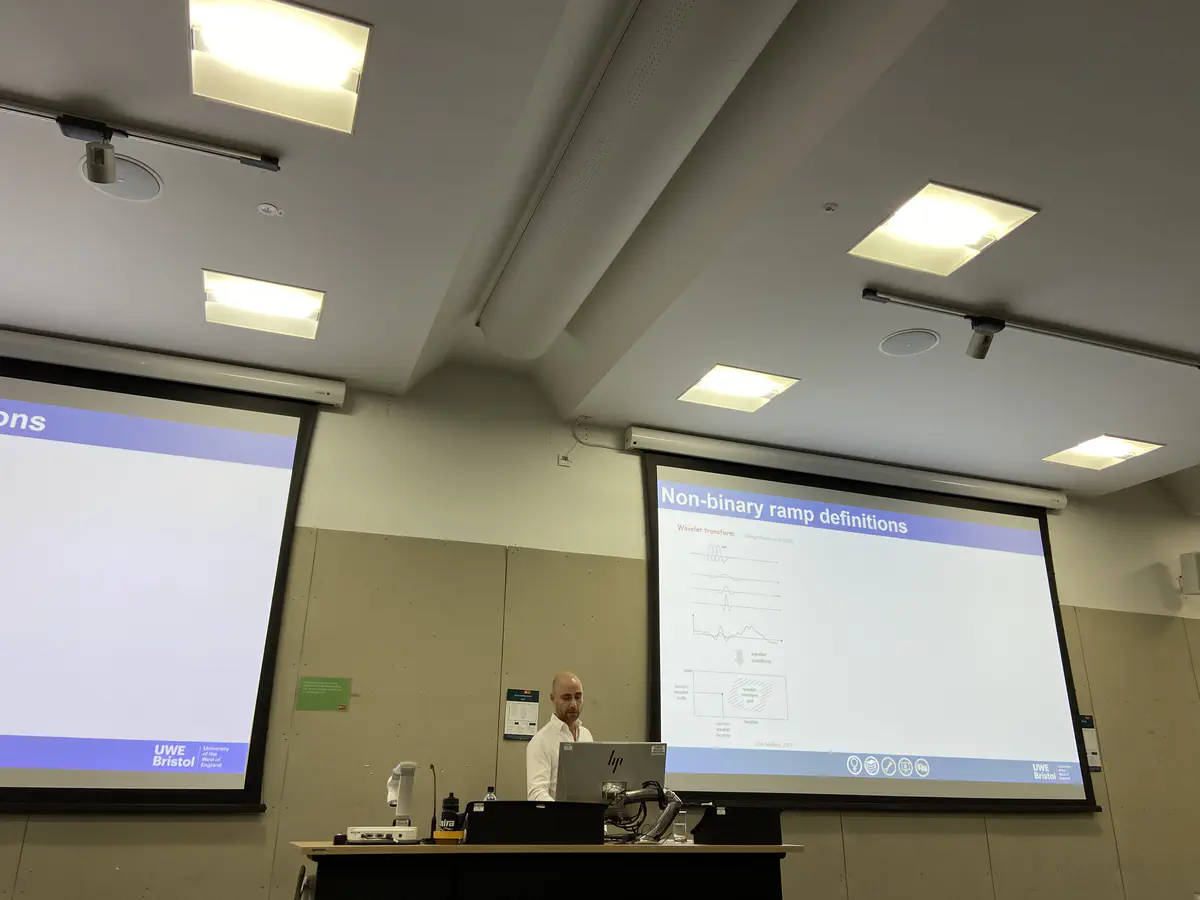
3International Conference for Sustainable Ecological Engineering Design for Society, SEEDS 2022.
Russell Sharp, my MSc Data Science Student, presented his thesis work titled “Integrating wind variability to modelling wind-ramp events using a non-binary ramp function and deep learning models” at the International Conference for Sustainable Ecological Engineering Design for Society, SEEDS 2022. See thesis (document) and a short video presentation below.
Abstract: The forecasting of large and rapid changes in wind power output known as ramp events is crucial for the incorporation of large volumes of wind energy into national electricity grids. Large variations in wind power supply must be compensated by ancillary energy sources which can include fossil fuels along with their associated costs and carbon emissions. Improved prediction of wind power will help to improve the competitiveness of wind energy against non-renewable energy sources, enable the global transition to cleaner fuels and facilitate climate change mitigation. This paper investigates the potential application of Machine Learning methodologies to the task of ramp event prediction. Using a non-binary wavelet transform approach to ramp characterisation, the ability of three Machine Learning models to predict ramp events in the Engie La Haute Borne wind farm dataset is compared. Results indicate that Recurrent Neural Network models show the greatest potential for ramp event forecasting.
-
👉 Conference details
-
📚 Thesis Preview
- 📚 Watch a brief summary of Sharp’s work:
Interested?
Please get in touch if you’d like exploring further work and development on wind power modelling and prediction.